The Future of Mathematics Education: Using Artificial Intelligence to Provide Efficient Feedback

This blog post was written by Cristina Heffernan and Dr. Hilary Kreisberg. If you would like to watch the full conference talk at NCTM click here.
Artificial Intelligence (AI) is all around you:
The GPS mapping tool you use on your phone may notice that the route you are on will take longer today due to an accident or road construction, so it offers another route for you.
We now have driverless cars that are using human intelligence without a human!
AI also writes our emails for us: Google has a Smart Reply tool that analyzes the emails you receive and provides suggested responses to make your reply quicker and easier.
Artificial intelligence also has applications for improving educational outcomes and the power to enhance teachers’ instructional practices.
We are Cristina Heffernan (Co-Founder of ASSISTments) and Dr. Hilary Kreisberg (Director of the Lesley University’s Center for Mathematics Achievement). We presented at the NCTM 100 Days of Professional Learning on the role of artificial intelligence in the future of math education, and specifically on how ASSISTments uses AI to support teachers in providing efficient feedback to students and scoring open response questions in mathematics. We've adapted our talk in this blog post to share ways in which we’ve been using AI to advance mathematics education.

As you can see from the image above, AI is made up of many parts. A new feature currently under development at ASSISTments makes use of two specific AI components: Machine Learning and Natural Language Processing.
What do we mean by this?
Machine learning (ML) allows computer systems use to perform a task without explicit instructions. One important aspect of ML is the data set the machine uses to learn from. For example, if you want a computer to be able to read handwritten numbers, you first need to provide it with a big set of human-developed examples. Then, the machine’s learning algorithms use what they see in that data set to try to do the task themselves.
Natural Language Processing (NLP) is the training of machines to read and derive meaning from human languages. For example, translation apps use NLP to read one language and then translate it into another.
The team at ASSISTments, along with Hilary’s team of mathematics education experts at Lesley University’s Center for Mathematics Achievement, have been working on a tool that, similar to Google’s Smart Reply feature for your email, helps teachers reply to students’ responses to open-ended questions with personalized and targeted feedback. We call it QUICK-Comments.
As with all of our features, our goals are to:
- enhance the ability of a teacher to reach their students with the supports they need
- reduce the time that it takes teachers to give students usable feedback and a score on their open response questions.
As math curricula encourage students to explain their thinking, we see many questions requiring open responses. Any teacher will tell you that open response questions are crucial for stimulating different types of mathematical reasoning, yet they also take a long time to read, score, and give feedback on. QUICK-Comments applies artificial intelligence to the scoring and feedback of these open response questions to make it quicker and easier for teachers.
Teachers are great at supporting and guiding their students. Machines are great at gathering and interpreting data. QUICK-Comments creates synergy between the two.
QUICK-Comments in Action
Curious what this will look like and how it will work? Let’s look at a Grade 6 math problem from the open source curriculum EngageNY, along with four student responses to the second part of the question, “how do you know?”
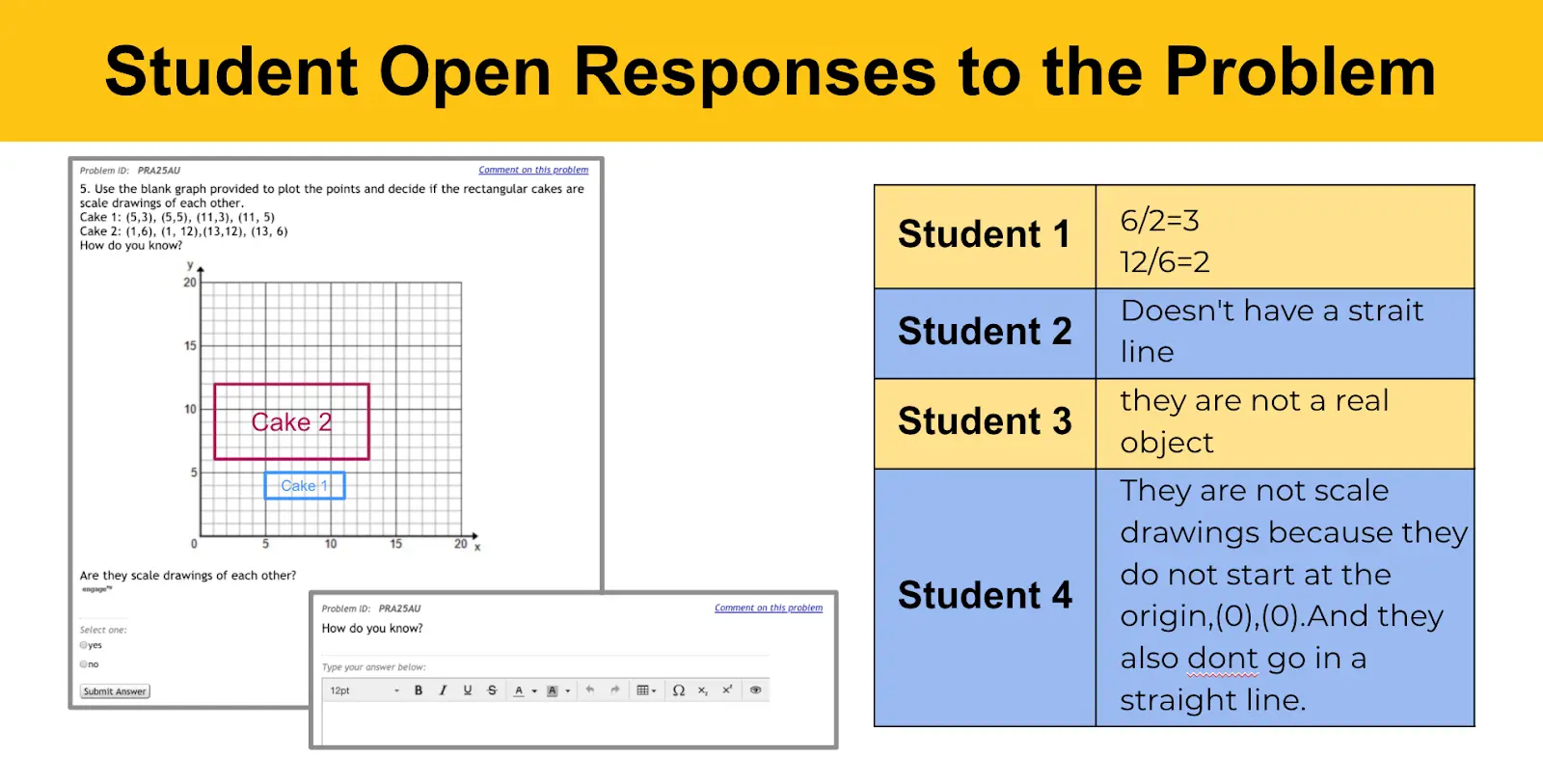
Right away, it’s clear that each student takes a very different approach to presenting their responses. Without QUICK-Comments, teachers must interpret the response and offer written feedback and/or a score. It currently looks like this:

Teachers read the responses, provide a score, and write as little or as much feedback as they’d like to each individual student. You can imagine how long this takes, especially for middle school teachers with multiple classes and up to 150 students! If a teacher assigns two open response questions four nights a week to 150 students, that’s 1200 responses a teacher has to read through and respond to individually. Yikes!
With QUICK-Comments, teachers will be able to preview suggested messages (that have been generated by teachers) that the machine has learned to apply to specific scores. Teachers simply choose from three options or write their own feedback message to student responses. It will look like this:
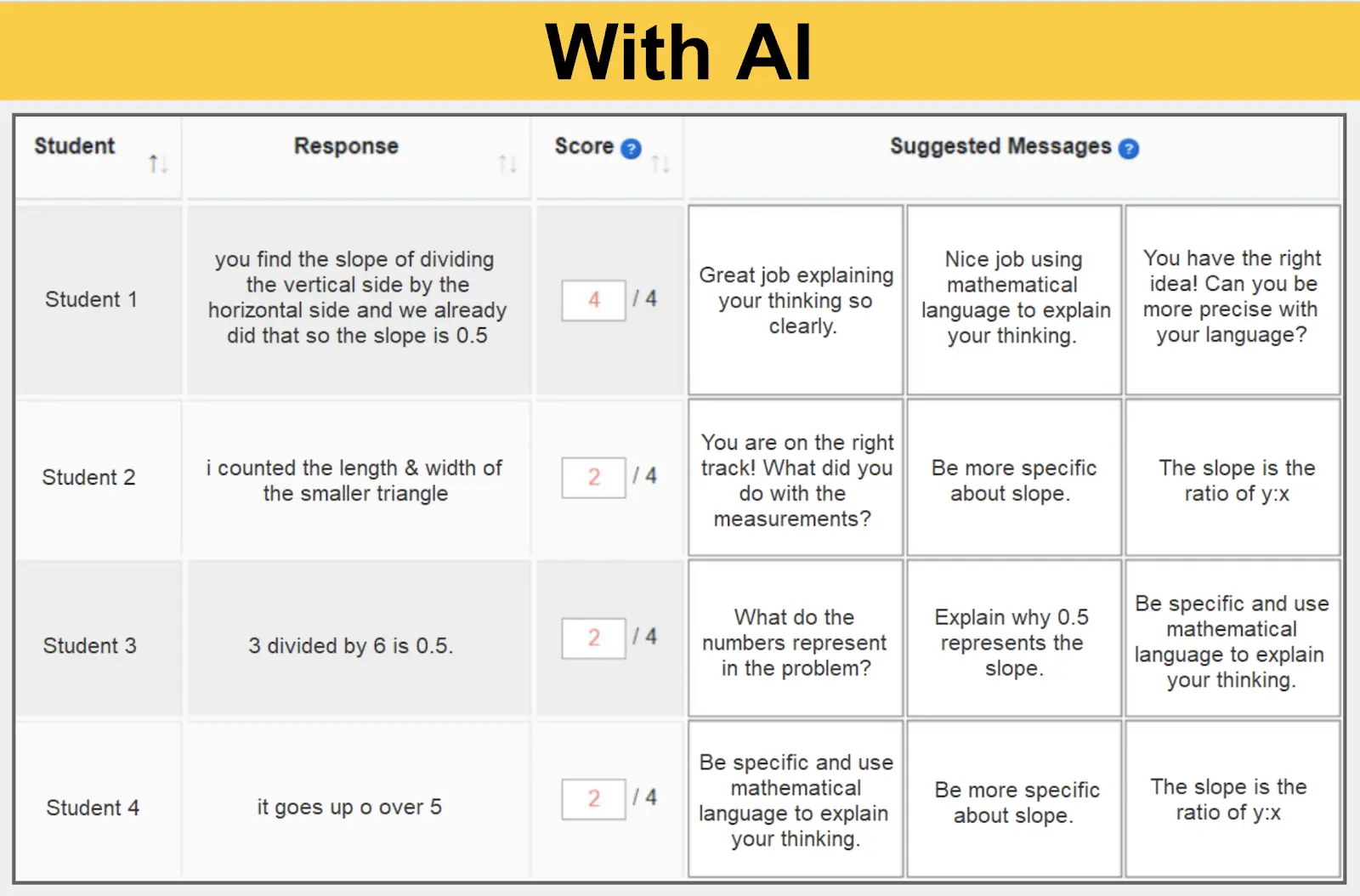
This makes the review and feedback process for teachers easier, but still keeps them in the driver’s seat. Teachers can keep or change the score, select, and use as is or augment a comment and send to students.
In our pilot tests, 100% of the teachers using the tool have noted that it has reduced the amount of time they spent scoring and providing feedback and made it easier for them to focus more on other instructional components of teaching.
Like with many tools that utilize AI methods, the more teachers use the feature, the more the machine learns, and the more effective the feedback.
Are you excited about the solutions QUICK-Comments can offer?
This feature is still in development, and not yet available in ASSISTments. We hope to make it available for full release soon, but if you want to join focus groups to test it out, please contact us and sign up to be a part of our Teachers for Research and Feedback community. We are excited for more teachers to try out our prototype and give feedback on how it will be useful in class.